A big congratulations to our residents: Nguyen Tuan Hai Dang, Vu Le The Anh, Nguyen Dinh Quoc, and former resident Nguyen Minh Huy who have had 3 papers published at the top-tier conference Machine Learning: International Conference on Machine Learning (ICML) 2022.
1. Improving Mini-batch Optimal Transport via Partial Transportation
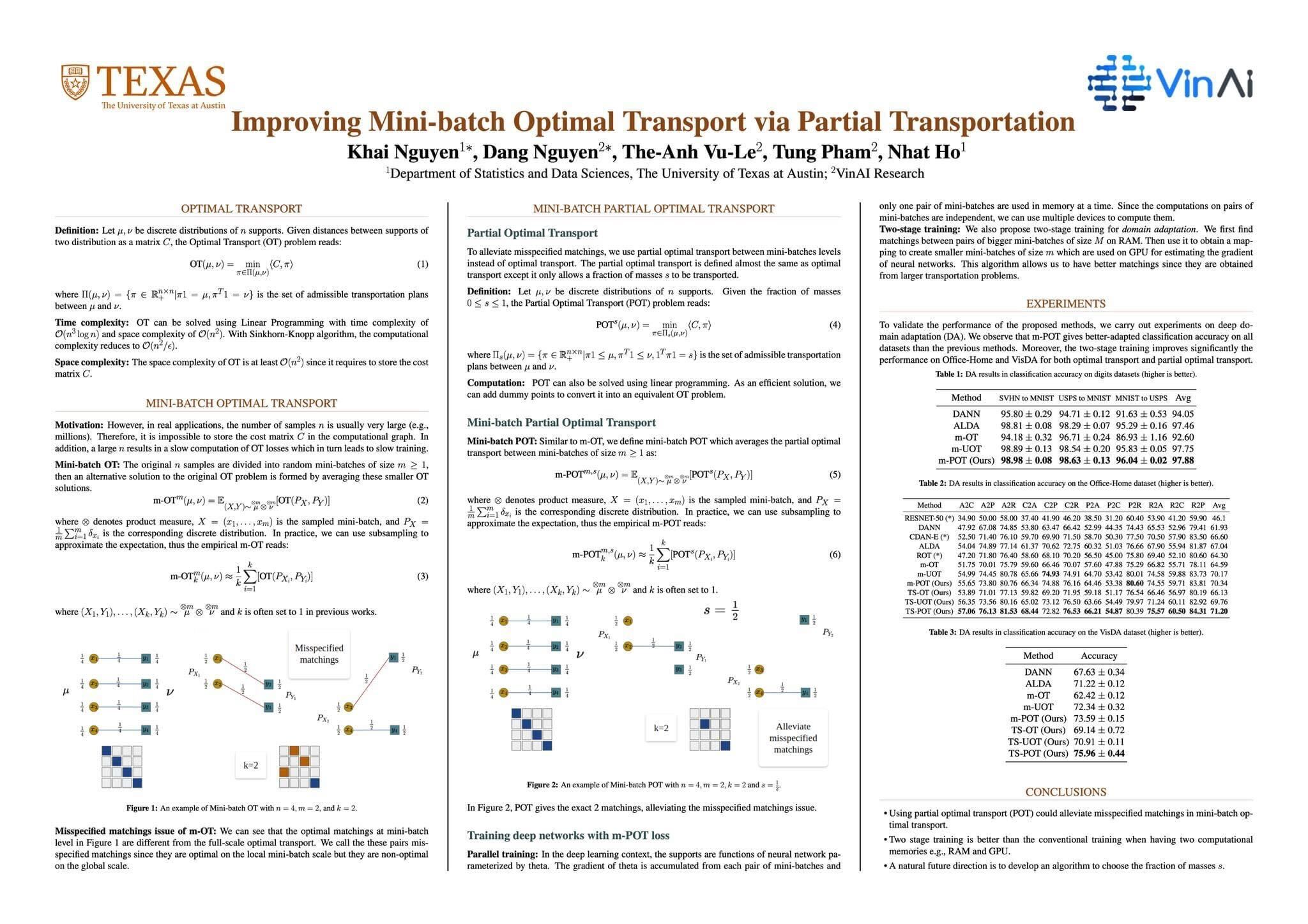
- Paper link: https://proceedings.mlr.press/v162/nguyen22e/nguyen22e.pdf
- Abstract: Mini-batch optimal transport (m-OT) has been widely used recently to deal with the memory issue of OT in large-scale applications. Despite their practicality, m-OT suffers from misspecified mappings, namely, mappings that are optimal on the mini-batch level but are partially wrong in the comparison with the optimal transportation plan between the original measures. Motivated by the misspecified mappings issue, we propose a novel mini-batch method by using partial optimal transport (POT) between mini-batch empirical measures, which we refer to as mini-batch partial optimal transport (m-POT). Leveraging the insight from the partial transportation, we explain the source of misspecified mappings from the m-OT and motivate why limiting the amount of transported masses among mini-batches via POT can alleviate the incorrect mappings. Finally, we carry out extensive experiments on various applications such as deep domain adaptation, partial domain adaptation, deep generative model, color transfer, and gradient flow to demonstrate the favorable performance of m-POT compared to current mini-batch methods.
2. On Transportation of Mini-batches: A Hierarchical Approach
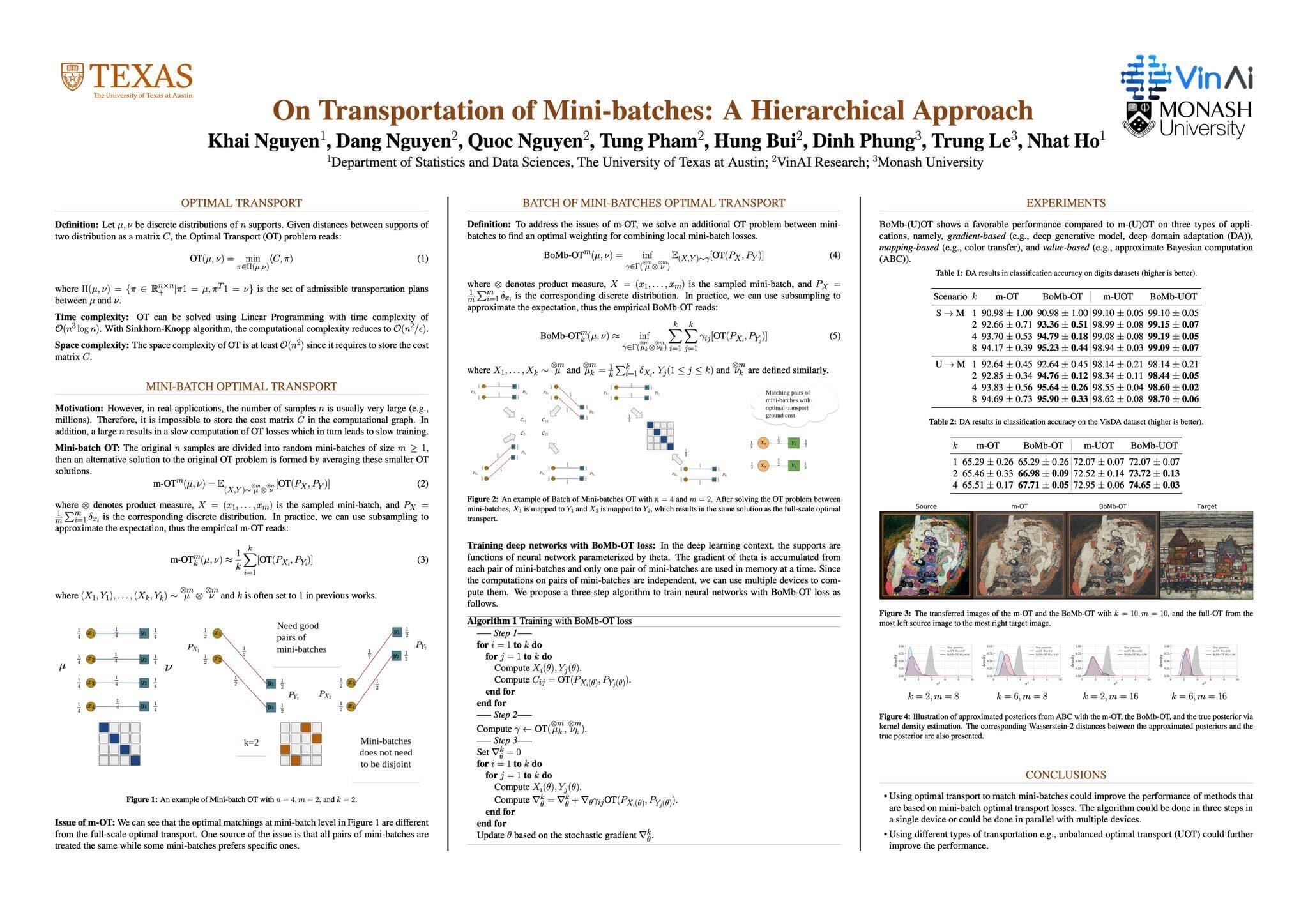
- Abstract: Mini-batch optimal transport (m-OT) has been successfully used in practical applications that involve probability measures with a very high number of supports. The m-OT solves several smaller optimal transport problems and then returns the average of their costs and transportation plans. Despite its scalability advantage, the mOT does not consider the relationship between mini-batches which leads to undesirable estimation. Moreover, the m-OT does not approximate a proper metric between probability measures since the identity property is not satisfied. To address these problems, we propose a novel mini-batch scheme for optimal transport, named Batch of Mini-batches Optimal Transport (BoMb-OT), that finds the optimal coupling between mini-batches and it can be seen as an approximation to a welldefined distance on the space of probability measures. Furthermore, we show that the m-OT is a limit of the entropic regularized version of the BoMb-OT when the regularized parameter goes to infinity. Finally, we carry out experiments on various applications including deep generative models, deep domain adaptation, approximate Bayesian computation, color transfer, and gradient flow to show that the BoMb-OT can be widely applied and performs well in various applications.
3. Entropic Gromov-Wasserstein between Gaussian Distributions
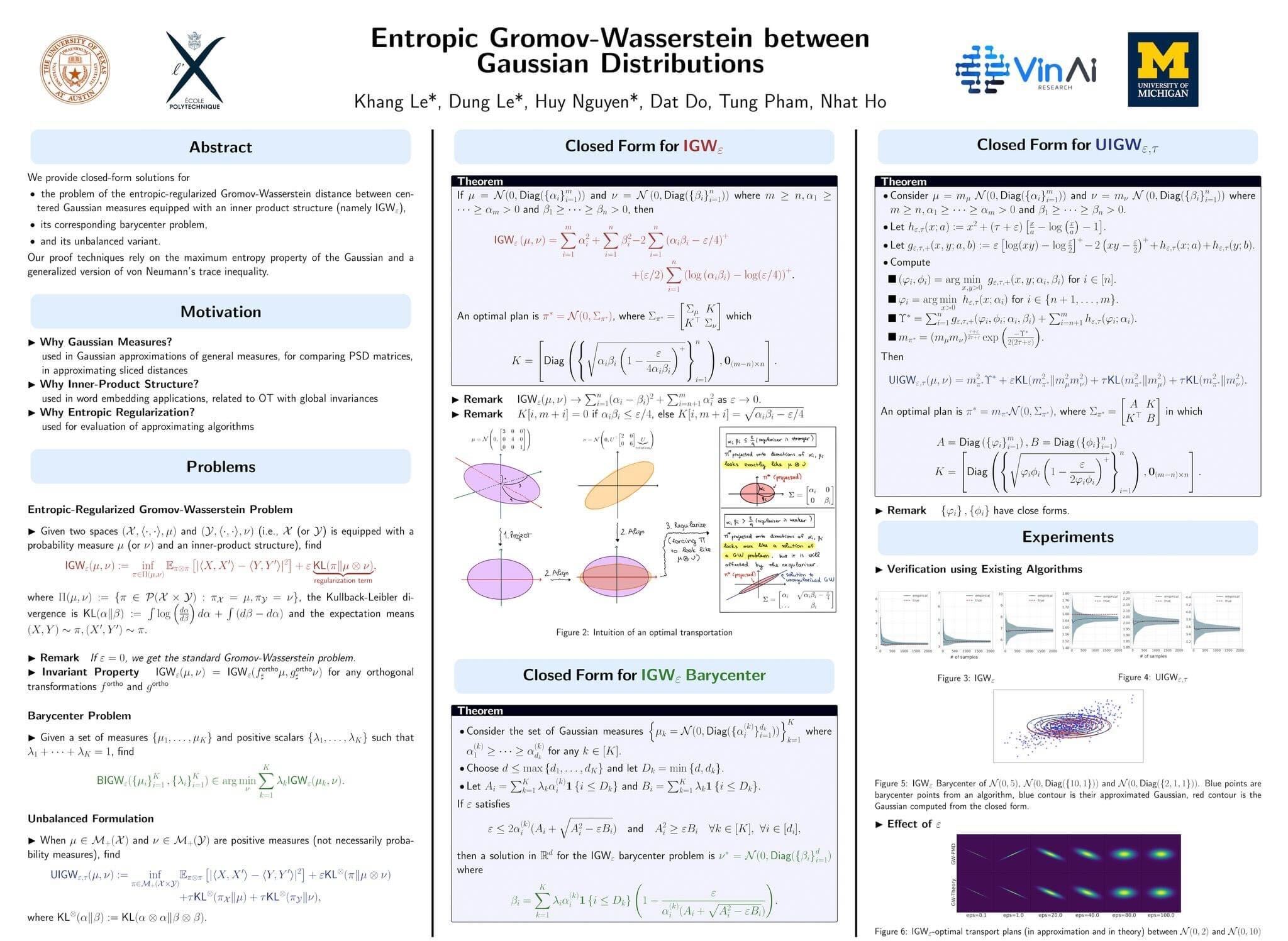
- Paper link: https://proceedings.mlr.press/v162/le22a/le22a.pdf
- Abstract: We study the entropic Gromov-Wasserstein and its unbalanced version between (unbalanced) Gaussian distributions with different dimensions. When the metric is the inner product, which we refer to as inner product Gromov-Wasserstein (IGW), we demonstrate that the optimal transportation plans of entropic IGW and its unbalanced variant are (unbalanced) Gaussian distributions. Via an application of von Neumann’s trace inequality, we obtain closed-form expressions for the entropic IGW between these Gaussian distributions. Finally, we consider an entropic inner product Gromov-Wasserstein barycenter of multiple Gaussian distributions. We prove that the barycenter is a Gaussian distribution when the entropic regularization parameter is small. We further derive a closed-form expression for the covariance matrix of the barycenter.
ICML is a premier conference for presenting and publishing cutting-edge research on various aspects of machine learning such as AI, statistics & data science, computational biology, machine vision, robotics and speech recognition.
In 2022, the conference was held from the 17 – 23 July, 2022 at the Baltimore Convention Center.